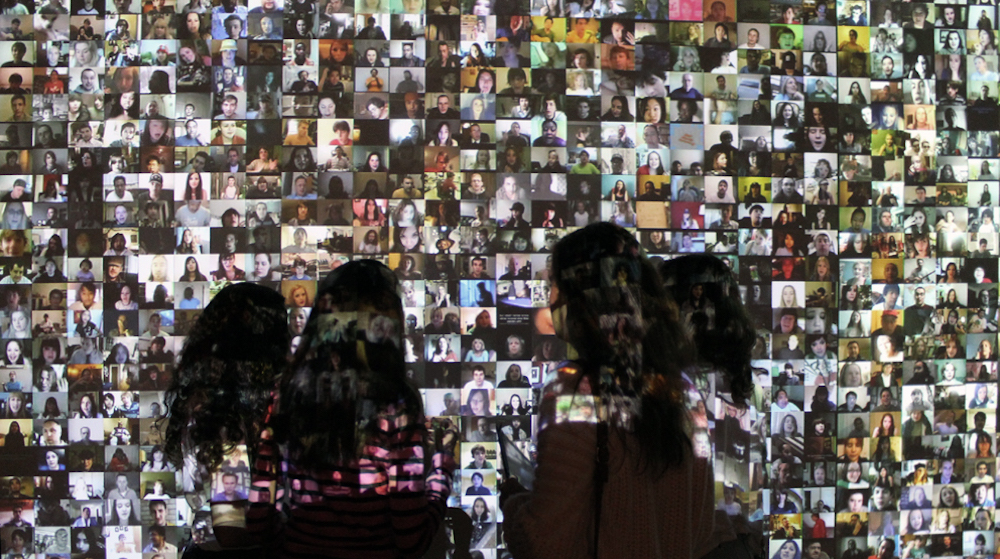
Donald Trump wants to ban all Muslims from entering the United States and has called for a wall to keep out Mexicans, whom he has called rapists and criminals. Many have called Trump a racist for these views because he lumps all members of a group—good and bad—together. But whether you admit it or not, you are probably somewhat racist too. We all are, to some extent. (If you’re shaking your head, there’s a nifty online test to remind you of your implicit biases.)
It may not be your fault. You are hardwired to be biased. The reason is that you have to make decisions based on limited information. Some of these decisions may determine whether you live or die. It’s important, for example, to make a snap judgment about whether that twisted sinister shape you see is a deadly poisonous snake or merely a rope. If you’re walking late one evening in a secluded, dimly lit neighborhood and you discern someone walking behind you, you need to figure out whether you might be attacked, robbed of your possessions, or molested. You must decide quickly if you should quicken your pace, change your direction, or even reach for the bottle of mace you are carrying. You are likely to make these decisions based on the stranger’s gender, race, age, or other physical characteristics. The same snap judgments must be made by policemen on the beat, often with tragic consequences, as we have witnessed in the past few years.
Such profiling scenarios raise several disturbing questions. Why do we tend to associate the color of one’s skin or other physical characteristics with the likelihood that you are in danger of being attacked? The answer is that we’ve learned to make associations using patterns and statistical data. But the underlying data available to each of us are very limited, and detectable signals we perceive quickly in making a probabilistic estimate are rather coarse. But we are trying to do the best we can. In other words, we use stereotypes—or small data. And when we act based on stereotypes and small data, we often discriminate—often unfairly, unjustly, and unkindly.
Most of us, of course, would prefer not to discriminate against people by placing them in groups and assigning average group characteristics to each individual in that large group. And so, even if we do it subconsciously, we admonish against stereotyping by telling ourselves, and anyone who will listen, that “Not all Muslims are terrorists,” “Not all Chinese drive slow,” and “Some white men can jump.”
But ignoring (small) data or statistical patterns that have predictive powers can be harmful. Sometimes it is practical to assume any snake you see on a hike is poisonous, and ascertain if you were right later on, after taking evasive action.
But our survival instincts, as well as good business practices, want us to make good predictions, not discriminate unnecessarily. If we had access to a lot of data, and could process large amounts of data quickly and efficiently, we would use all data available to us in making predictions. And we could see which data points turn out to be the most critical.
Most online retailers, such as Amazon, do not care per se what your gender or ethnicity is, how old you are, and whether or not you are rich. They merely want to predict, based on your browsing and purchasing behavior, what they can sell to you next. So, for instance, Amazon might assume that if you purchased all the books or movies in The Hunger Games series, you’ll likely enjoy the Divergent series, and it’s basing that assumption on observed behavior, regardless of your race, age, or gender. The more data Amazon has on your buying patterns and preferences, the more accurate its customer segmentation algorithms will prove, and the more the retailer can treat you like an individual, instead of as a stereotype. That is the promise of big data.
This raises the question of if and how big data can help the rest of us in other situations. For example, could we leverage big data to solve the problem of the stranger walking behind us? Will there be some sci-fi solution where our phone scans for everyone around and then your smartwatch will give you the threat assessment using a green, yellow, or red alert based on your own level of fear and paranoia? Advances in wearable sensor technology could detect patterns in the way the stranger moves to determine danger, without violating anyone’s privacy through use of personal data. For example, observing the movements of a person behind you may reveal if you are being followed closely, or if he is carrying a weapon with him, or if he is lurching at you to attack you. Such technology already exists, and is being deployed for so-called “deep learning paradigms” being developed for self-driving cars.
Of course, big data may also be used to create more stereotypes. Big data is already guilty of stereotyping people based on where they live (see this article in the Harvard Business Review by Michael Schrage). For example, customers living in certain urban zip codes may be classified, often unjustly, as being more “difficult,” best avoided by national businesses choosing where to expand their reach. Further research is needed to understand whether big data will generate more stereotypes than it will eradicate.
Our conjecture—and we are currently testing it with formal research—is that once we have easy access to a large number of characteristics and variables and we can crunch all that data with greater speed and with greater accuracy, the coarse signals such as race, gender, ethnicity, and sexual orientation will become redundant in making useful predictions about survival and well-being. Furthermore, even if the coarse signals do have marginal value in making useful predictions, a regulatory ban or societal censure discouraging use of stereotypes will not impose a significant cost. Our research will examine data on bank loans to determine if a set of borrower attributes can be used to predict defaults and delinquencies adequately, at the same time making sure that these very attributes do not correlate with race, gender, ethnicity, or other discriminatory stereotypes.
Big data has immense potential to trump stereotypes derived from small data. Human intelligence, which is limited and has small computing power, will be supplanted by AI devices (we’d call them robots, but that makes some of you squeamish) with immense computing power, which will allow us to make more efficient decisions while becoming more enlightened, tolerant, and kind to our fellow human beings. In other words, big data can address the legitimate concerns underlying Donald Trump’s policies, while trumping the racism they trigger.
Send A Letter To the Editors